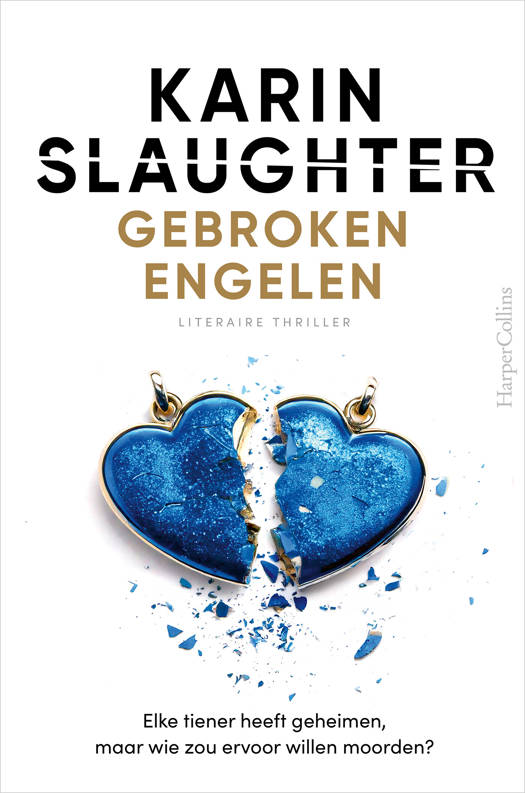
Markov decision process (MDP) models are widely used for modeling sequential decision-making problems that arise in engineering, economics, computer science, and the social sciences. This book provides practical modeling methods for many real-world problems with high dimensionality or complexity which have not hitherto been treatable with Markov decision processes. In addition to providing numerous specific algorithms, coverage includes both illustrative numerical examples and rigorous theoretical convergence results. The algorithms developed and analyzed differ from the successful computational methods for solving MDPs based on neuro-dynamic programming or reinforcement learning and will complement work in those areas. In addition, the book shows how to combine the various algorithms introduced with approximate dynamic programming methods that reduce the size of the state space and ameliorate the effects of dimensionality.
We publiceren alleen reviews die voldoen aan de voorwaarden voor reviews. Bekijk onze voorwaarden voor reviews.