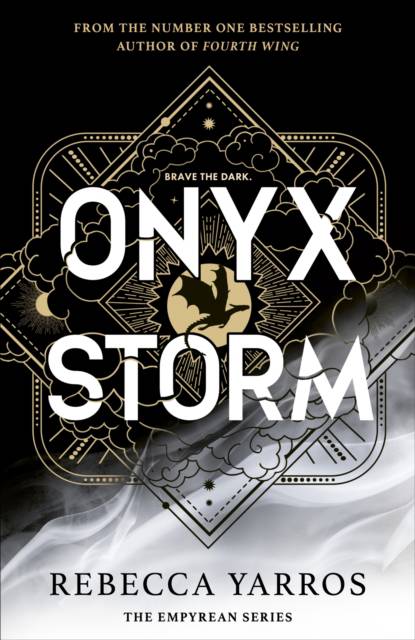
Regularization, Optimization, Kernels, and Support Vector Machines offers a snapshot of the current state of the art of large-scale machine learning, providing a single multidisciplinary source for the latest research and advances in regularization, sparsity, compressed sensing, convex and large-scale optimization, kernel methods, and support vector machines. Consisting of 21 chapters authored by leading researchers in machine learning, this comprehensive reference:
Regularization, Optimization, Kernels, and Support Vector Machines is ideal for researchers in machine learning, pattern recognition, data mining, signal processing, statistical learning, and related areas.
We publiceren alleen reviews die voldoen aan de voorwaarden voor reviews. Bekijk onze voorwaarden voor reviews.