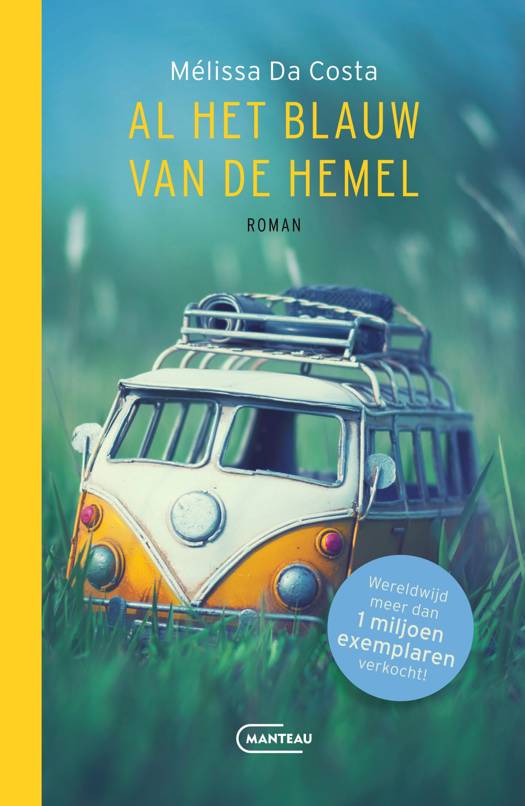
This book introduces an optimal iterative learning control (ILC) design framework from the end user's point of view. Its central theme is the understanding of model dynamics, the construction of a procedure for systematic input updating and their contribution to successful algorithm design. The authors discuss the many applications of ILC in industrial systems, applications such as robotics and mechanical testing.
The text covers a number of optimal ILC design methods, including gradient-based and norm-optimal ILC. Their convergence properties are described and detailed design guidelines, including performance-improvement mechanisms, are presented. Readers are given a clear picture of the nature of ILC and the benefits of the optimization-based approach from the conceptual and mathematical foundations of the problem of algorithm construction to the impact of available parameters in making acceleration of algorithmic convergence possible. Three case studies on robotic platforms, an electro-mechanical machine, and robot-assisted stroke rehabilitation are included to demonstrate the application of these methods in the real-world.
With its emphasis on basic concepts, detailed design guidelines and examples of benefits, Optimal Iterative Learning Control will be of value to practising engineers and academic researchers alike.
We publiceren alleen reviews die voldoen aan de voorwaarden voor reviews. Bekijk onze voorwaarden voor reviews.