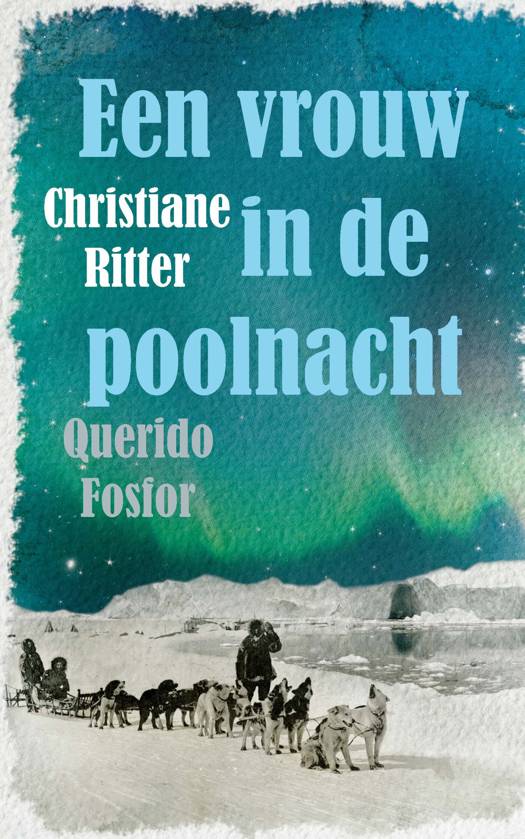
This book presents an in-depth exploration of multimodal learning toward recommendation, along with a comprehensive survey of the most important research topics and state-of-the-art methods in this area.
First, it presents a semantic-guided feature distillation method which employs a teacher-student framework to robustly extract effective recommendation-oriented features from generic multimodal features. Next, it introduces a novel multimodal attentive metric learning method to model user diverse preferences for various items. Then it proposes a disentangled multimodal representation learning recommendation model, which can capture users' fine-grained attention to different modalities on each factor in user preference modeling. Furthermore, a meta-learning-based multimodal fusion framework is developed to model the various relationships among multimodal information. Building on the success of disentangled representation learning, it further proposes an attribute-driven disentangled representation learning method, which uses attributes to guide the disentanglement process in order to improve the interpretability and controllability of conventional recommendation methods. Finally, the book concludes with future research directions in multimodal learning toward recommendation.
The book is suitable for graduate students and researchers who are interested in multimodal learning and recommender systems. The multimodal learning methods presented are also applicable to other retrieval or sorting related research areas, like image retrieval, moment localization, and visual question answering.
We publiceren alleen reviews die voldoen aan de voorwaarden voor reviews. Bekijk onze voorwaarden voor reviews.